Multimodal architectures are revolutionizing the way systems process and interpret complex data. These advanced architectures facilitate simultaneous analysis of diverse data types such as text and images, broadening AI’s capabilities to mirror human cognitive functions more accurately. The seamless integration of these modalities is crucial for developing more intuitive and responsive AI systems that can…
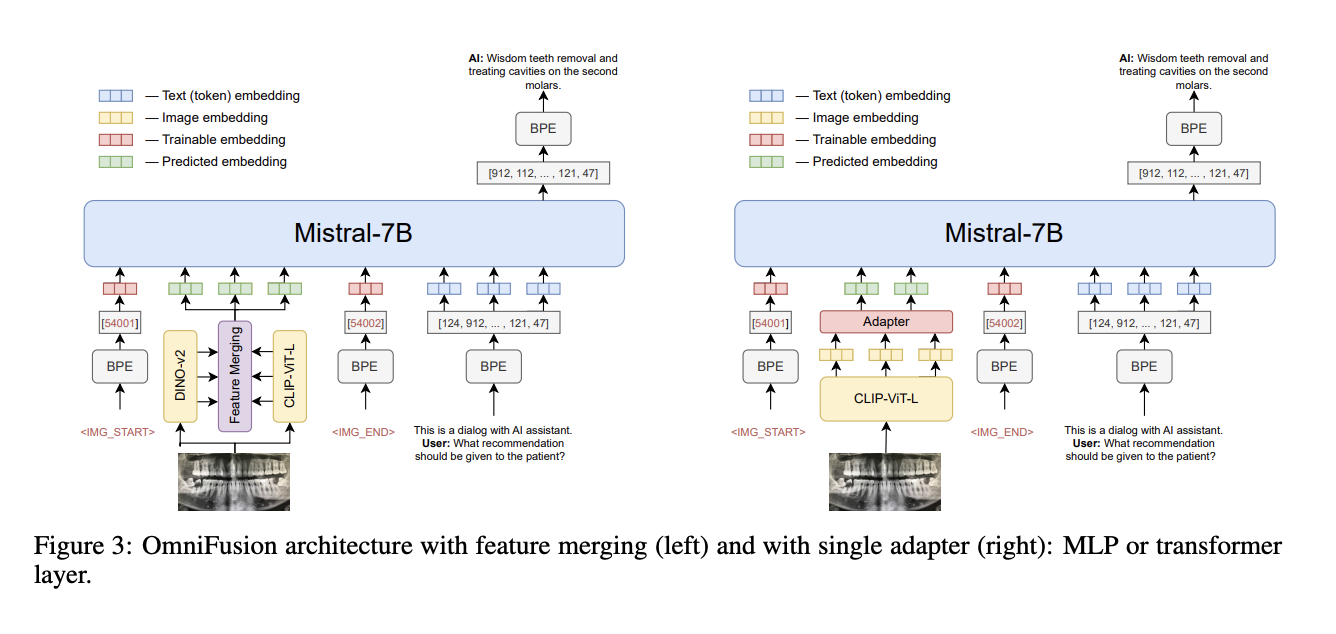