3D occupancy estimation methods initially relied heavily on supervised training approaches requiring extensive 3D annotations, which limited scalability. Self-supervised and weakly-supervised learning techniques emerged to address this issue, utilizing volume rendering with 2D supervision signals. These methods, however, faced challenges, including the need for ground truth 6D poses and inefficiencies in the rendering process. Existing…
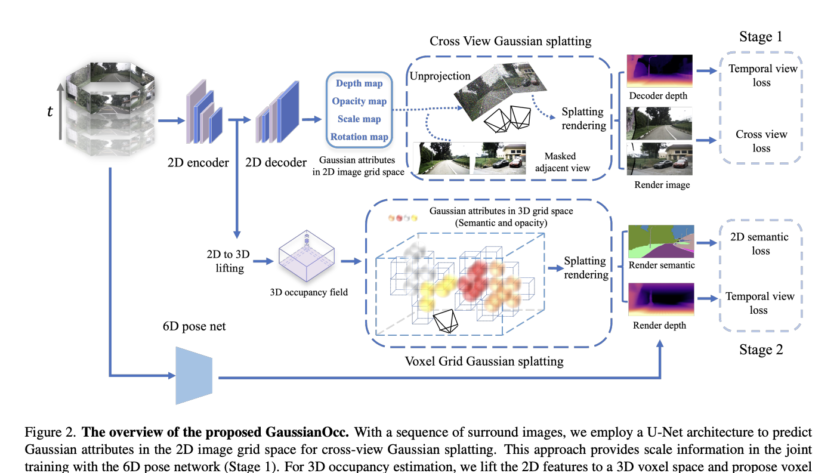