Data workflows are essential for businesses to make informed decisions and drive growth. However, managing these workflows can be time-consuming and repetitive, often involving manual tasks that drain valuable resources.
Businesses often end up spending a lot of time on data preparation and cleaning, leaving little room for strategic analysis. While these tasks are necessary, they do not best use your team’s skills.
Automating data workflows isn’t a new concept, but recent advancements in intelligent automation solutions have made it possible to streamline even the most complex processes. You can now automate data extraction, integration, and analysis, enabling your team to focus on high-value activities and deliver meaningful results faster.
In this guide, we’ll explore best practices, real-world examples, and powerful tools to help you automate your data workflows and get more out of your data.
What is a data workflow?
A data workflow is a structured process for combining data from various sources and formats into an integrated data set. Its main goal is to provide users with a structured, complete view of data to support confident decision-making across the enterprise.
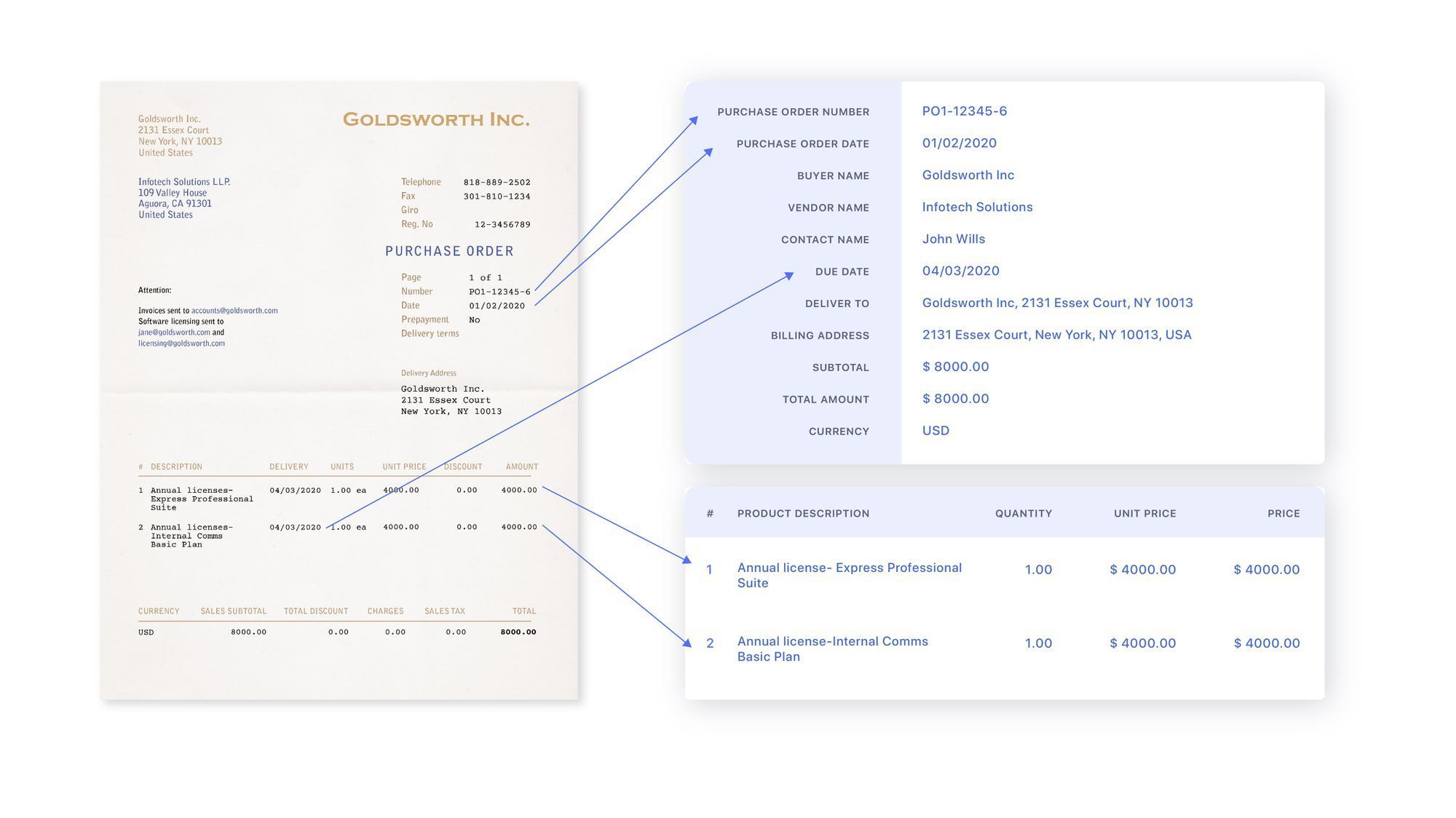
To ensure the accuracy and integrity of the process and the resulting analysis, those tasked with managing data workflows typically follow a step-by-step approach:
- Identifying and collecting data for inclusion: Ensures the inclusion of relevant data that will meet the end user’s needs.
- Cleansing data: Preliminary analysis is used to identify and address data quality issues such as missing data, duplicate records, data with questionable provenance, and records that contain errors.
- Standardization and normalization: Ensures the data uses the same format, structure, and scale to facilitate subsequent analysis.
- Data assurance: Creating and applying data quality standards to improve data quality, including a remediation process to amend or remove data to improve overall quality.
- Data storage: This involves identifying locations to store data sets and selecting the storage format and tools to facilitate analysis and reporting.
- Data analysis: In the final step, analysts use methodologies, tools, and techniques to analyze the data and extract findings that support robust decision-making.
Data workflow examples
Using a data integration workflow allows analysts to develop an all-encompassing view of the data, aggregated data from different sources. This further enables the enterprise to make confident, data-driven decisions.
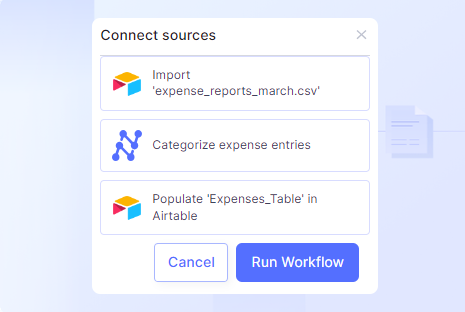
Here are some examples of data workflows in various industries:
- Integrating customer data from multiple touchpoints into a single database
- Automating data extraction from financial statements, contracts, and other documents for analysis and reporting
- Syncing customer data between CRM, order management, and support systems
- Automating invoice processing, approval, and payment
- Monitoring cash flow and generating compliance reports
- Integrating patient data from EHRs, lab results, and devices for clinical decision support
- Integrating data from inventory, logistics, and supplier systems
- Automating customer order processing and fulfillment based on real-time inventory data
Why you need to optimize your data workflows
Inefficient data workflows can significantly hinder your business. Manual tasks, scattered data sources, and inconsistent processes lead to wasted time, costly errors, and missed opportunities.
But when you streamline your data workflows, amazing things happen:
- Save time and boost productivity: Automated data workflows eliminate manual tasks and repetitive work. No more copying and pasting data between systems or chasing down missing information. Your team can focus on high-value activities that drive results.
- Improve data quality and consistency: Efficient data workflows ensure data is captured, cleaned, and standardized at every step. Say goodbye to data entry errors, duplicates, and inconsistencies that can skew your analysis and lead to bad decisions.
- Enable faster, better decision-making: Clean, reliable data allows you to make informed decisions quickly. Automating data workflows for financial reporting provides real-time visibility into cash flow, expenses, and profitability. Integrating data from CRM, marketing, and support systems gives you a comprehensive view of your customers, enabling data-driven decisions to improve retention and growth.
- Scale your data operations: As your business grows, so does your data. Optimized data workflows can handle increasing volumes and complexity without missing a beat. You’ll be able to onboard new data sources, automate more processes, and efficiently support more users.
- Gain a competitive edge: Businesses that can harness their data effectively win. Efficient data workflows give you the agility and insights to outmaneuver competitors, seize opportunities, and delight customers.
- Free up your team to focus on high-value work: Automating data workflows frees your team to focus on strategic work by reducing manual data tasks. For instance, automating expense report processing allows your finance team to concentrate on financial planning, while automating data preparation enables analysts to spend more time uncovering insights and recommending actions.
The bottom line? Efficient data workflows are not just a nice-to-have—they’re a must for businesses that want to stay agile, competitive, and customer-centric.
To get started, identify your business’s most inefficient and error-prone data workflows, such as manual data entry or reconciliation. Then, explore user-friendly, scalable, and secure tools like intelligent document processing and data integration platforms to automate and optimize those workflows. Lastly, involve your team, communicate the benefits, and provide training to ensure successful adoption.
How to streamline your data workflows
Businesses must now process vast amounts of information quickly and accurately to stay competitive. Streamlining data workflows is crucial to achieving this goal. However, with data’s increasing volume and complexity, manual processing is no longer feasible.
This is where intelligent automation comes in. By leveraging advanced technologies such as Optical Character Recognition (OCR), Artificial Intelligence (AI), Machine Learning (ML), Robotic Process Automation (RPA), and integrations, businesses can automate and optimize their data workflows from end to end.
Quick overview of these technologies:
- Optical Character Recognition (OCR) converts images of text into machine-readable data
- Artificial Intelligence (AI) and Machine Learning (ML) enable computers to understand, analyze, and learn from data
- Robotic Process Automation (RPA) automates repetitive, rule-based tasks
- Integrations and APIs allow different systems to communicate and exchange data seamlessly
Nanonets is an AI-powered document processing platform that helps businesses streamline their data workflows by automating data capture, classification, validation, enrichment, integration, processing, and analytics. With Nanonets, organizations can process structured and unstructured documents faster, more accurately, and at scale, enabling them to make data-driven decisions and focus on growing their business.
To get started, look for repetitive tasks that take a lot of time but don’t require human decision-making. For example, if your team spends hours manually extracting data from invoices each day, that’s a clear opportunity for automation.
Now, let’s explore how these technologies can streamline each step of your data workflow:
1. Data capture
OCR technology can automatically extract data from scanned documents, images, or PDFs, eliminating the need for manual data entry. Nanonets’ OCR engine can accurately capture data from structured and unstructured documents, such as invoices, forms, and receipts.
Example: Automatically capture invoice numbers, dates, and totals from supplier invoices
2. Data classification
AI and ML algorithms can analyze and classify the extracted data based on predefined categories or rules. Nanonets uses advanced AI and ML models to automatically classify documents and extract relevant information, such as vendor names, invoice numbers, and amounts.
Example: Route documents to the appropriate workflow based on their type, such as invoices to accounts payable and purchase orders to procurement.
3. Data validation
Automated data validation ensures the accuracy and completeness of the extracted data. Nanonets can validate data against predefined rules or external databases, flagging any.
Example: Flag invoices with missing or invalid PO numbers discrepancies for manual review. This reduces errors and ensures data integrity.
4. Data enrichment
AI and ML can help enrich the extracted data with additional information from internal or external sources. Nanonets can automatically transform your data by integrating with your existing systems or third-party APIs, providing a more comprehensive view of your data.
Example: Match supplier names from invoices with vendor records in your ERP system to ensure consistency and accuracy.
5. Data integration
Integrations and APIs enable the automated transfer of data between different systems, eliminating the need for manual data entry and reducing errors. Nanonets seamlessly integrates with your existing business applications, such as ERP, CRM, and accounting software, allowing you to push or pull data between systems automatically.
Example: Push extracted and enriched customer data to your CRM system to keep customer records up-to-date and enable personalized marketing campaigns.
6. Data processing
RPA can automate repetitive, rule-based tasks in your data workflow, such as formatting, mapping, and entry. Nanonets provides a flexible, no-code platform for designing and automating your data processing tasks, enabling you to streamline your workflows without technical expertise.
Example: Automatically format extracted data to match your system’s requirements, such as converting date formats or mapping data fields.
7. Data analytics
AI and ML can help analyze the processed data to uncover insights, patterns, and trends. Platforms like Nanonets provide powerful analytics and reporting capabilities, allowing you to gain real-time visibility into your data and make informed decisions based on accurate, up-to-date information.
Example: By analyzing invoice data, businesses can identify spending patterns, optimize vendor relationships, and improve cash flow management.
Final thoughts
While not every step of a data workflow can or should be automated, identifying repetitive, time-consuming tasks is critical to optimizing your process. By leveraging intelligent tools and platforms like Nanonets, you can streamline data capture, validation, enrichment, and integration, saving valuable time and resources. This lets your team focus on what matters most – analyzing insights, making informed decisions, and driving business success.
As data volumes grow, efficiently managing and utilizing data will become increasingly critical. Adopting best practices and embracing automation can set your organization up for success in today’s data-driven world. Start small, iterate often, and continuously look for opportunities to optimize your data workflows. The benefits – from increased productivity to better decision-making – are worth the effort.