In the ever-evolving domain of remote identification technologies, gait recognition stands out for its unique capacity to identify individuals from a certain distance without requiring direct engagement. This cutting-edge approach leverages the distinctive walking patterns of each person, offering a seamless integration into surveillance and security systems. Its non-intrusive nature distinguishes it from more conventional…
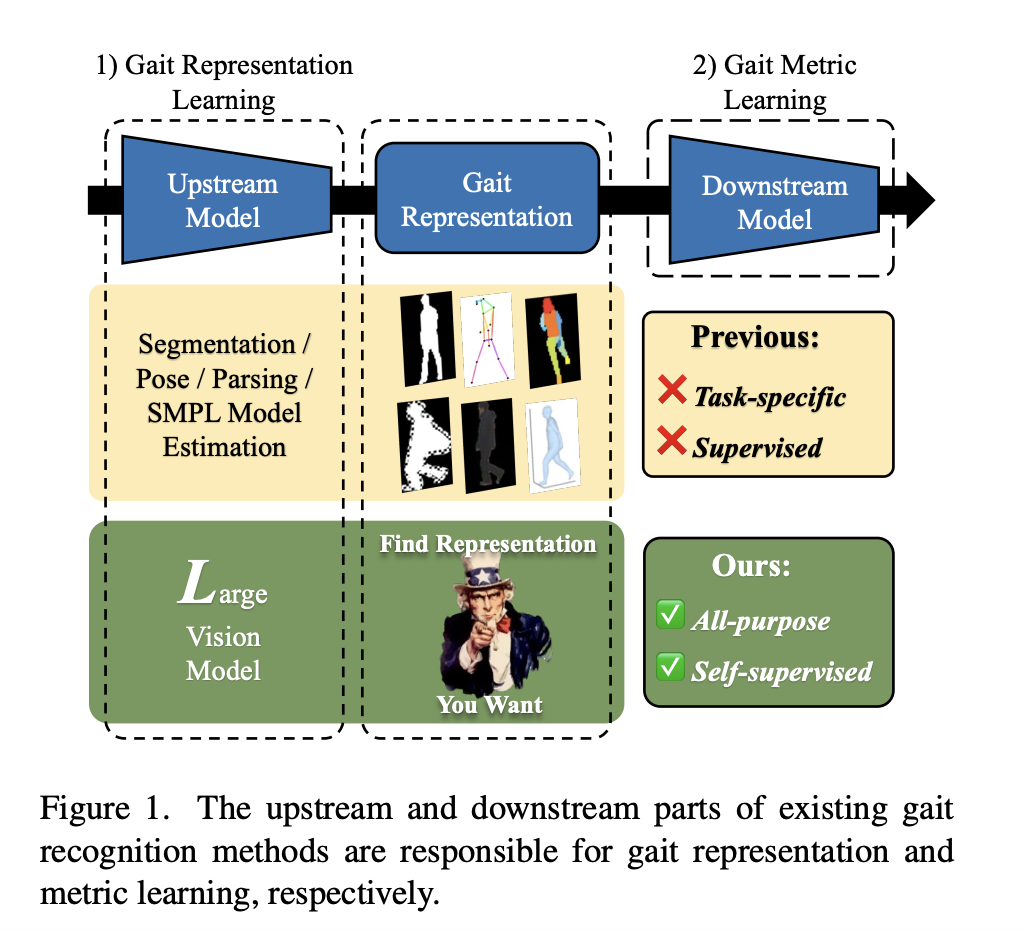